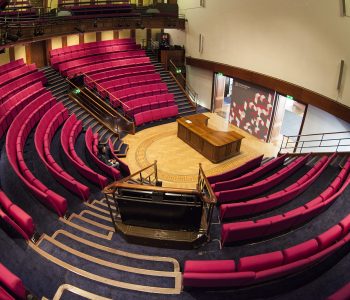
It’s unwise to guess lecture room sizes
The University of the North reluctantly decided that it had to imitate its rivals and open a London satellite campus (UNLS). A search for suitable premises led to a lease on four floors of Mistral House, a commercial building of the 1970s in a central but affordable location.
Read more “It’s unwise to guess lecture room sizes”